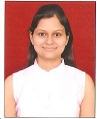 |
|
ANKITA GUPTA |
|
|
|
Master of Technology
In
Information Systems
|
Year of Publish -2016 |
Month - February |
|
|
|
|
|
|
Personal Multimodal Biometric Authentication Using Unsupervised Learning, Hidden Markov Model (HMM) |
7 |
Under Guidence of : - Sh. Anil Singh Parihar |
Email : - *****shikha.akhil.singhal@gmail.com****
|
Master of Technology
In
Information Systems |
|
Abstract
Biometric authentication systems have been used since decades. Palmprint and finger knuckle prints are two such modalities that are universal and possess uniqueness. A variety of algorithms are available to extract features from these modalities and do the authentication process. In this report, use of a machine learning, unsupervised Hidden Markov Model algorithm is proposed to classify the users into genuine and imposter classes. In the following report, a multimodal system using palmprint and finger knuckle print has been proposed using a combination of Harris Corner Detector; SIFT descriptors and Continuous Density Hidden Markov Model (CDHMM). Here the states defining the origination of the observation feature vectors are hidden. The features are extracted using Harris Corner Detector and are described using Scale Invariant Feature Descriptor (SIFT). An approach is proposed to do the authentication at feature level as well as at score level. The log-likelihood computed by HMM and the parameters are maximised by Expectation-Maximization Algorithm. An iterative approach is used to increase the authentication rates and to get the correct number of states in each Hidden Markov Model of each user at feature level and for genuine and imposter classes at score level. The various fusion methods at score level are experimented for the PolyU, IITD palmprint and PolyUfinger knuckle print database. The authentication rates obtained are as high as 99% GAR at 0.01 FAR forPolyUpalmprint database that are comparable to other methods of authentication at score level. The highest GAR was recorded using SUM fusion rule. The authentication rates are high for feature level authentication as well for both knuckle prints and palmprints. GAR was recorded as high as 97% for right middle knuckle finger print at 0.01 FAR.
|